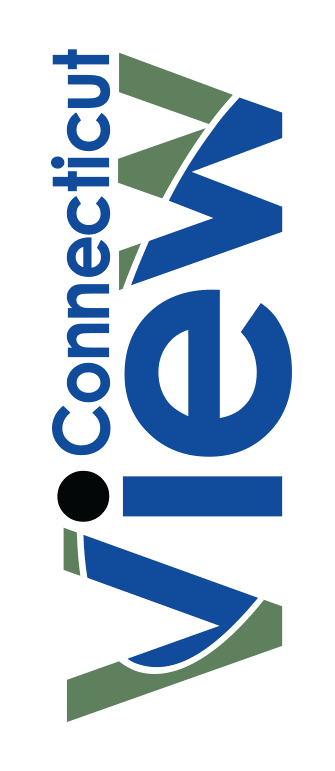
- ESA Sentinel-2A Earth Observation Satellite
- Landsat 8 OLI Image of southeastern Connecticut showing gypsy moth defoliation, image captured June 27, 2016.
- Sentinel-2 false color image of UConn main campus, Storrs, Connecticut. Image captured March 23, 2021
- A Normalize Difference Vegetation Index (NDVI) map of UConn main campus, Storrs, Connecticut. Sentinel-2 image captured March 23, 2021
DISTRIBUTION OF INVASIVE JAPANESE BARBERRY AND MULTIFLORA ROSE IN CONNECTICUT
The goal of this study is to determine the value of producing statewide maps for Japanese Barberry (Berberis thunbergii) and Multiflora Rose (Rosa multiflora) utilizing medium resolution satellite imagery from Sentinel-2/Landsat. Early spring is the best period to use remote sensing imagery to monitor both populations, according to their distinctive phenological signals using normalized difference vegetation index (NDVI). With the extended leaf phenology of invasive plants, researchers can distinguish them from native plants before the upper canopy closes using early spring (leaf-off) images.
|
Drone video of UConn Fenton Tract showing understory plants leafing out before the canopy closes in mid-May, 2018. Drone video credit: Dr. Nancy Marek, Founder of BirdHabitatBot. |
In this study, we created a Multinomial Logistic Regression (MLR), which is a machine learning based approach, to map two invasive plant species using Sentinel-2 time series imagery. To train and validate the model, we are working closely with a variety of stakeholders, including Connecticut DEEP (Department of Energy and Environmental Protection), CIPWG (Connecticut Invasive Plant Working Group), UConn forestry extension, UConn agricultural experiment station, land trusts, and other conservation organizations. Maps of invasive plant species will be shared as ArcGIS StoryMaps. A GitHub repository will make training data, machine learning models, and open-source pipelines, available to multiple users.
![]() |
NDVI map of the UConn Campus in Storrs, CT. Sentinel-2 image acquired on March 23, 2021 |
We studied the response of seven vegetation indices, corresponding to 36 study sites, according to the occurrence of three vegetation types during period when invasive shrubs show the extended plant leaf phenology. Training data was built from available scenes from early March to late May. The data consists of Sentinel-2 image scenes acquired from 2018-2023. The objectives of the analysis were to identify: (1) the best time for distinguishing the three vegetation types and (2) which indices would be most important to accurately determine the probability of a pixel being an invasive understory pixel due the spread of Berberis thunbergii or/and Rosa multiflora infestations in Connecticut forests. The Distribution map can be viewed here.
![]() |
Responses of different vegetation indices for early springtime at the UConn Forest Study Sites. Sentinel-2 BOA values were used to build the boxplots. Class 1 = Invasive pixels, 2 = Deciduous and 3= Evergreen. The time period from early March to Late May has been divided into biweekly phases, which are named from phase 01 to phase 06. |
CONNECTICUT SURFACE WATER CLARITY ASSESSMENT (MULTI-TEMPORAL ASSESSMENT OF CONNECTICUT LAKE WATER CLARITY)
Fresh water bodies are one of the important features of the natural resources in the Connecticut which provide habitat for wildlife, drinking water, and recreation. Eutrophication is one of the alarming challenges, that Connecticut experiences. Eutrophication is a natural or a man-made scenario which happens due to the accumulation of excessive amounts of nutrients like nitrogen and cyanobacterial blooms are one of the visual indicators of the phenomenon. Since 2004 till the present, the Connecticut Agricultural Experiment Station (CAES) has conducted surveys of lakes throughout CT. Connecticut's first cyanobacterial bloom was noted in 2012. In this study, a device known as a secchi disk is used by the Connecticut Agriculture Experiment Station to gauge the transparency of water. The secchi disk measures depth by first being lowered into the water until it is no longer discernible. The disk is then raised again via the water column. A second depth is determined after the disk is once more visible. Then, the average of the two depths is determined. These metrics aid in determining a body of water's general clarity.
The goal of this project was to have a complete assessment of Connecticut's lakes. Using satellite imagery provides a big picture of statewide lake and pond water quality by enhancing in situ measurements. In this method, water pixels were found to be spectrally distinct from most other land cover features. Pixel groups were designated and classified as belonging to the water category or not. The pixel clusters were recoded into water (class 1) and non-water (class 0). (class 1). The water pixels are subjected to a clump method to determine clusters of nearby water pixels that correspond to waterbody features. To create a feature layer of waterbodies larger than three acres, water clumps with an extent of less than three acres are sieved out of the clump layer. The original Landsat image's water pixels are extracted using this waterbody feature layer and employed in the regression model process. Final data products include Connecticut statewide water clarity estimates for the months June – September (one water clarity estimation per month), for the years 2015 – 2020. It was possible to better pinpoint potential nutrient input sources by examining the spatial distribution of algae and eutrophication inside lakes. Finding of this study will help establishing a lake water quality monitoring system to determine which lakes and ponds may need more urgent research and management to reduce impacts on water quality.
CONNECTICUT GYPSY MOTH CATERPILLAR FOREST DEFOLIATION 2016, 2017, and 2018
Researchers at ConnecticutView have developed Gypsy Moth caterpillar forest defoliation extent maps for Connecticut for the years 2016, 2017, and 2018. These maps were created through difference analysis of Enhanced Vegetation Indices derived from Landsat 8 and Sentinel-2 satellite imagery.Maps and images may be downloaded in the Research section in the Navigation tabs above.
CONGRATULATIONS
to Zhiyaun Zang, a 2016 ConnecticutView Undergraduate Scholarship receipient on the publication of his paper,”Using Landsat 8 data to compare percent impervious surface area and normalized difference vegetation index as indicators of urban heat island effects in Connecticut, USA“, in the journal Environmental Earth Sciences.
Yang, Z., Witharana, C., Hurd, J. et al. Using Landsat 8 data to compare percent impervious surface area and normalized difference vegetation index as indicators of urban heat island effects in Connecticut, USA. Environ Earth Sci 79, 424 (2020). https://doi.org/10.1007/s12665-020-09159-0
PURPOSE
USGS Land Remote Sensing Program
- An error has occurred, which probably means the feed is down. Try again later.
Quick Links
Contact Us
Phone: | (860) 486-8732 |
---|---|
E-mail: | chandi.witharana@uconn.edu |
Address: | Unit 4087 1376 Storrs Rd. Storrs, CT 06269-4087 |